How to Use Data Analytics to Improve Your Marketing Efforts
What Is Data Analytics and Why Is It Important for Marketing?
Data analytics refers to the systematic computational analysis of data or statistics. In marketing, it involves using data-driven insights to inform and refine strategies to achieve better outcomes. This practice is crucial because it allows marketers to make informed decisions rather than relying on intuition alone. By leveraging data, businesses can understand consumer behaviour, track campaign performance, and optimise marketing strategies.
The Role of Data Analytics in Marketing
- Consumer Insight: Data analytics helps in understanding customer preferences, behaviours, and demographics. By analysing this data, marketers can tailor their messages to specific audience segments, making campaigns more relevant and engaging.
- Campaign Performance: Tracking and analysing campaign data provides insights into what is working and what is not. This allows for the optimisation of current campaigns and the formulation of more effective future strategies.
- Trend Identification: By examining trends and patterns in data, marketers can anticipate market changes and adapt strategies accordingly. This proactive approach can give businesses a competitive edge.
Key Components of Data Analytics in Marketing
- Data Collection: Gathering data from various sources such as social media, website analytics, and customer feedback is the first step. This data forms the foundation for analysis.
- Data Cleaning: Raw data often contains inconsistencies and errors. Data cleaning ensures that the data used for analysis is accurate and reliable.
- Data Analysis: Using statistical tools and software, marketers analyse the cleaned data to uncover patterns and insights. Techniques such as regression analysis and clustering are commonly used.
- Reporting and Visualisation: Presenting data in a comprehensible manner through reports and visualisations helps stakeholders understand the insights and make data-driven decisions.
- Implementation: Applying the insights gained from data analysis to marketing strategies to improve effectiveness and achieve better results.
How Can Data Analytics Help Identify Target Audiences?
Identifying target audiences is a crucial aspect of marketing that data analytics significantly enhances. By analysing customer data, businesses can segment their audience into distinct groups based on various criteria such as demographics, behaviour, and preferences.
Methods to Identify Target Audiences Using Data Analytics
- Segmentation: Data analytics allows for segmentation based on demographic factors (age, gender, income) and psychographic factors (lifestyle, values). This helps in creating targeted marketing campaigns for each segment.
- Behavioural Analysis: By examining customer behaviour such as purchase history, browsing patterns, and interaction with marketing materials, businesses can identify patterns and preferences, allowing for more precise targeting.
- Predictive Analytics: Using historical data and statistical models, predictive analytics can forecast future behaviour and trends. This helps in anticipating the needs of different audience segments and tailoring marketing strategies accordingly.
- Customer Journey Mapping: Data analytics can track the entire customer journey from initial contact to conversion. Understanding this journey helps in identifying key touchpoints and optimising marketing efforts for different stages.
- Lookalike Audiences: By analysing characteristics of existing customers, businesses can create profiles of lookalike audiences. These are potential customers who share similar traits with current high-value customers, making them ideal targets for marketing campaigns.
Tools and Techniques for Audience Analysis
- CRM Systems: Customer Relationship Management (CRM) systems collect and analyse data on customer interactions and transactions. This information is valuable for understanding customer needs and preferences.
- Google Analytics: This tool provides insights into website visitors, including demographics, interests, and behaviour. It helps in identifying high-performing audience segments.
- Social Media Analytics: Platforms like Facebook and Twitter offer analytics tools that provide data on user interactions and engagement. This helps in understanding audience preferences and behaviour on social media.
- Surveys and Feedback: Collecting feedback directly from customers through surveys helps in gaining insights into their needs and preferences.
- Data Visualization Tools: Tools such as Tableau and Power BI help in visualising audience data, making it easier to identify patterns and trends.
What Are the Best Practices for Data-Driven Marketing Strategies?
Implementing data-driven marketing strategies requires a structured approach to ensure effectiveness. Following best practices ensures that data is used efficiently to achieve marketing goals.
Best Practices for Data-Driven Marketing
- Define Clear Objectives: Establish clear marketing objectives that align with business goals. This helps in focusing data analysis on relevant metrics and KPIs.
- Collect Relevant Data: Ensure that data collected is relevant to the objectives. Avoid data overload by focusing on metrics that directly impact marketing performance.
- Ensure Data Quality: High-quality data is essential for accurate analysis. Implement data cleaning processes to eliminate errors and inconsistencies.
- Use Advanced Analytics Tools: Leverage advanced analytics tools and software to gain deeper insights. Tools like Google Analytics, SEMrush, and HubSpot offer valuable features for data analysis.
- Personalise Marketing Efforts: Use data insights to personalise marketing messages and offers. Personalisation enhances customer engagement and increases conversion rates.
- Monitor and Adjust: Continuously monitor marketing performance and adjust strategies based on data insights. Regularly review KPIs and metrics to ensure alignment with objectives.
- Integrate Data Sources: Integrate data from various sources to get a comprehensive view of customer behaviour and marketing performance. This helps in making informed decisions.
- Train Your Team: Ensure that your marketing team is well-trained in data analytics and interpretation. Provide training and resources to enhance their data-driven decision-making skills.
- A/B Testing: Conduct A/B testing to compare different marketing strategies and determine which performs better. This helps in optimising campaigns based on data-driven results.
- Stay Updated: Data analytics and marketing technologies are constantly evolving. Stay updated with the latest trends and tools to maintain a competitive edge.
How Can Data Analytics Improve Customer Retention?
Customer retention is vital for long-term business success, and data analytics plays a significant role in improving it. By analysing customer data, businesses can implement strategies to enhance satisfaction and loyalty.
Strategies to Improve Customer Retention Using Data Analytics
- Personalised Communication: Use customer data to personalise communication and offers. Tailoring messages to individual preferences increases engagement and loyalty.
- Predictive Analytics: Implement predictive analytics to identify customers at risk of churn. By anticipating potential issues, businesses can take proactive measures to retain these customers.
- Customer Feedback Analysis: Regularly analyse customer feedback to identify areas for improvement. Addressing concerns and making necessary changes enhances customer satisfaction.
- Loyalty Programs: Develop and optimise loyalty programs based on customer data. Analyse participation and reward preferences to create effective programmes that encourage repeat business.
- Segmentation: Segment customers based on behaviour and preferences to provide targeted retention strategies. Customising retention efforts for different segments increases effectiveness.
- Behavioural Triggers: Set up behavioural triggers for automated communication based on customer actions. For example, sending a follow-up email after a purchase can encourage repeat business.
- Churn Analysis: Conduct churn analysis to understand why customers leave. Identifying common factors helps in developing strategies to address and mitigate these issues.
- Customer Journey Analysis: Map the customer journey to identify key touchpoints and moments of truth. Enhancing these touchpoints improves overall customer experience and retention.
- Engagement Metrics: Monitor engagement metrics such as open rates, click-through rates, and interaction levels. Use these insights to adjust retention strategies and improve customer engagement.
- Cross-Selling and Upselling: Analyse purchase history to identify opportunities for cross-selling and upselling. Offering relevant products or services increases customer value and retention.
What Tools and Software Are Essential for Data Analytics in Marketing?
Data analytics tools and software are crucial for gathering, analysing, and interpreting data. Selecting the right tools enhances the effectiveness of data-driven marketing strategies.
Essential Tools and Software for Data Analytics
- Google Analytics: Provides comprehensive insights into website traffic, user behaviour, and conversion metrics. Essential for understanding online marketing performance.
- HubSpot: A CRM platform with powerful analytics features. It offers insights into marketing campaigns, lead generation, and customer interactions.
- Tableau: A data visualisation tool that helps in creating interactive and shareable dashboards. It simplifies the analysis of complex data sets.
- SEMrush: An SEO and digital marketing tool that provides insights into keyword performance, competitor analysis, and market trends.
- Power BI: A business analytics tool that enables data visualisation and reporting. It integrates with various data sources for comprehensive analysis.
- Adobe Analytics: Offers advanced analytics capabilities for tracking customer interactions across multiple channels. It helps in understanding customer behaviour and preferences.
- Social Media Analytics Tools: Tools like Hootsuite and Sprout Social provide insights into social media performance, engagement, and audience demographics.
- Survey Tools: Tools such as SurveyMonkey and Typeform help in collecting and analysing customer feedback. They provide valuable insights into customer needs and preferences.
- Marketing Automation Platforms: Platforms like Marketo and Pardot offer data-driven marketing automation features, including lead scoring and campaign tracking.
- Customer Data Platforms (CDPs): CDPs consolidate customer data from various sources into a unified view. They help in personalising marketing efforts and improving customer experience.
What Are Common Challenges in Implementing Data Analytics for Marketing?
While data analytics offers numerous benefits, implementing it can pose several challenges. Understanding these challenges helps in addressing them effectively.
Common Challenges in Data Analytics for Marketing
- Data Quality Issues: Poor data quality can lead to inaccurate insights. Ensuring data accuracy through cleaning and validation is essential for reliable analysis.
- Data Overload: The sheer volume of data can be overwhelming. Focusing on relevant metrics and using effective data management practices helps in managing data overload.
- Integration Difficulties: Integrating data from various sources can be complex. Using tools and platforms that support data integration simplifies the process.
- Skill Gaps: A lack of skilled personnel can hinder data analysis efforts. Investing in training and hiring qualified professionals helps in overcoming skill gaps.
- Privacy Concerns: Data privacy regulations and concerns can impact data collection and usage. Ensuring compliance with privacy laws and best practices is crucial.
- Cost: Implementing data analytics tools and technologies can be expensive. Assessing the return on investment and selecting cost-effective solutions helps in managing expenses.
- Complexity of Analysis: Advanced analytics techniques can be complex and require specialised knowledge. Using user-friendly tools and providing training helps in addressing this challenge.
- Resistance to Change: Resistance from stakeholders or team members can impede the adoption of data-driven strategies. Communicating the benefits and providing support helps in overcoming resistance.
- Real-Time Data Processing: Processing and analysing data in real-time can be challenging. Using real-time analytics tools and technologies helps in addressing this issue.
- Measurement of ROI: Measuring the return on investment for data analytics initiatives can be difficult. Establishing clear objectives and tracking relevant metrics helps in evaluating ROI.
How Do You Measure the Success of Data-Driven Marketing Campaigns?
Measuring the success of data-driven marketing campaigns involves evaluating various metrics and KPIs to determine effectiveness. Understanding these metrics helps in assessing performance and making improvements.
Metrics and KPIs for Measuring Success
- Conversion Rate: The percentage of visitors who take the desired action, such as making a purchase or signing up for a newsletter. A higher conversion rate indicates successful campaigns.
- Return on Investment (ROI): The ratio of net profit to the cost of the campaign. ROI measures the financial return generated by marketing efforts.
- Customer Acquisition Cost (CAC): The cost associated with acquiring a new customer. Lower CAC indicates efficient marketing strategies.
- Customer Lifetime Value (CLV): The total revenue generated from a customer over their lifetime. Higher CLV signifies successful retention strategies.
- Click-Through Rate (CTR): The percentage of users who click on a link or ad compared to the total number of viewers. A higher CTR indicates effective ad copy and targeting.
- Engagement Metrics: Metrics such as likes, shares, comments, and time spent on content. High engagement levels reflect successful content and audience connection.
- Lead Generation: The number of leads generated from marketing campaigns. Tracking lead quality and quantity helps in evaluating campaign effectiveness.
- Sales Growth: The increase in sales revenue attributable to marketing efforts. Tracking sales growth provides insights into the impact of marketing strategies.
- Website Traffic: The number of visitors to the website and their behaviour. Increased traffic and positive user behaviour indicate successful marketing efforts.
- Customer Feedback: Feedback and reviews from customers. Positive feedback indicates successful marketing and customer satisfaction.
What Are the Future Trends in Data Analytics for Marketing?
Staying informed about future trends in data analytics helps businesses to remain competitive and adapt to changes in the marketing landscape.
Emerging Trends in Data Analytics for Marketing
- Artificial Intelligence (AI) and Machine Learning: AI and machine learning technologies are increasingly used for predictive analytics, personalisation, and automating marketing tasks.
- Big Data: The growing volume of data presents opportunities for deeper insights and more sophisticated analytics. Big data technologies enable the processing of vast amounts of information.
- Real-Time Analytics: Real-time analytics provides immediate insights into marketing performance and customer behaviour, allowing for quick adjustments to strategies.
- Data Privacy: With increasing concerns about data privacy, businesses are focusing on complying with regulations and implementing privacy-conscious practices.
- Customer Data Platforms (CDPs): CDPs are becoming more prevalent, offering a unified view of customer data and enabling more effective personalisation and targeting.
- Voice Search Analytics: As voice search becomes more popular, analysing voice search data helps in understanding user intent and optimising content for voice queries.
- Visual and Video Analytics: The rise of visual and video content requires new analytics approaches to measure engagement and effectiveness.
- Integration of Marketing and Sales Data: Combining marketing and sales data provides a more comprehensive view of customer interactions and helps in aligning strategies.
- Augmented Reality (AR) Analytics: AR technology is being used to enhance customer experiences, and analytics are being developed to measure its impact.
- Advanced Attribution Models: New attribution models are being developed to more accurately measure the impact of various marketing channels and touchpoints.
How Can Small Businesses Leverage Data Analytics for Marketing?
Data analytics is not just for large enterprises; small businesses can also benefit from data-driven marketing strategies. Leveraging data effectively can help small businesses compete and grow.
Strategies for Small Businesses
- Start Small: Begin with basic analytics tools and gradually scale up as needed. Focus on key metrics that align with business goals.
- Use Free Tools: Take advantage of free or low-cost analytics tools such as Google Analytics and social media insights. These tools provide valuable data without significant investment.
- Leverage CRM Systems: Implement a CRM system to manage customer data and track interactions. This helps in understanding customer needs and preferences.
- Focus on Customer Feedback: Collect and analyse customer feedback to gain insights into satisfaction and areas for improvement.
- Monitor Competitors: Use analytics to monitor competitors’ performance and strategies. This helps in identifying opportunities and areas for differentiation.
- Personalise Marketing Efforts: Use data to personalise marketing messages and offers. Personalisation enhances customer engagement and loyalty.
- Track ROI: Measure the return on investment for marketing campaigns to ensure that resources are used effectively.
- Optimise Social Media: Analyse social media performance to understand what content resonates with the audience and adjust strategies accordingly.
- Invest in Training: Provide training for staff to enhance their data analysis skills. This improves the effectiveness of data-driven marketing efforts.
- Stay Agile: Be flexible and adapt strategies based on data insights. Regularly review performance and make adjustments to optimise results.
What Are the Ethical Considerations in Data Analytics for Marketing?
Ethical considerations are crucial in data analytics to ensure that practices are responsible and respectful of privacy.
Key Ethical Considerations
- Data Privacy: Ensure compliance with data privacy regulations such as GDPR and CCPA. Protecting customer data and obtaining consent is essential.
- Transparency: Be transparent with customers about data collection and usage practices. Clearly communicate how their data will be used and stored.
- Data Security: Implement robust security measures to protect data from breaches and unauthorised access. Regularly update security protocols.
- Avoiding Bias: Ensure that data analysis and algorithms do not perpetuate biases or discrimination. Regularly review and test algorithms for fairness.
- Responsible Use of Data: Use data responsibly and avoid manipulative or deceptive practices. Ensure that marketing efforts are honest and respectful.
- Consent: Obtain explicit consent from customers before collecting and using their data. Provide options for customers to opt-out if they choose.
- Data Accuracy: Ensure that data used for analysis is accurate and up-to-date. Avoid making decisions based on inaccurate or outdated information.
- Ethical AI: If using AI or machine learning, ensure that the technology is used ethically and does not infringe on privacy or rights.
- Customer Empowerment: Empower customers by providing them with control over their data and allowing them to access and correct their information.
- Regulatory Compliance: Stay informed about evolving data privacy laws and regulations. Ensure that data analytics practices comply with legal requirements.
Summary Table
Topic | Key Points |
---|---|
Data Analytics in Marketing | Systematic analysis of data to make informed decisions and optimise strategies. |
Identifying Target Audiences | Segmentation, behavioural analysis, predictive analytics, and customer journey mapping. |
Best Practices | Define objectives, ensure data quality, personalise efforts, and integrate data sources. |
Customer Retention | Personalisation, predictive analytics, feedback analysis, and loyalty programmes. |
Essential Tools | Google Analytics, HubSpot, Tableau, SEMrush, Power BI, and CRM systems. |
Challenges | Data quality issues, integration difficulties, privacy concerns, and cost. |
Measuring Success | Conversion rate, ROI, CAC, CLV, CTR, engagement metrics, and sales growth. |
Future Trends | AI, big data, real-time analytics, data privacy, CDPs, and voice search analytics. |
Small Businesses | Start small, use free tools, leverage CRM systems, and focus on customer feedback. |
Ethical Considerations | Data privacy, transparency, data security, avoiding bias, and obtaining consent. |
FAQ
What is data analytics in marketing?
Data analytics in marketing involves analysing data to understand consumer behaviour, track campaign performance, and optimise marketing strategies for better outcomes.
How can data analytics help identify target audiences?
Data analytics helps in identifying target audiences by segmenting customers, analysing behaviour, using predictive models, and mapping customer journeys.
What are the best practices for implementing data-driven marketing strategies?
Best practices include defining clear objectives, ensuring data quality, using advanced analytics tools, personalising marketing efforts, and integrating data sources.
How can data analytics improve customer retention?
Data analytics improves customer retention by personalising communication, using predictive analytics to identify churn risks, and optimising loyalty programmes.
What tools are essential for data analytics in marketing?
Essential tools include Google Analytics, HubSpot, Tableau, SEMrush, Power BI, and CRM systems.
What are common challenges in implementing data analytics for marketing?
Challenges include data quality issues, data overload, integration difficulties, skill gaps, and privacy concerns.
How do you measure the success of data-driven marketing campaigns?
Success is measured through metrics such as conversion rate, ROI, CAC, CLV, CTR, engagement metrics, and sales growth.
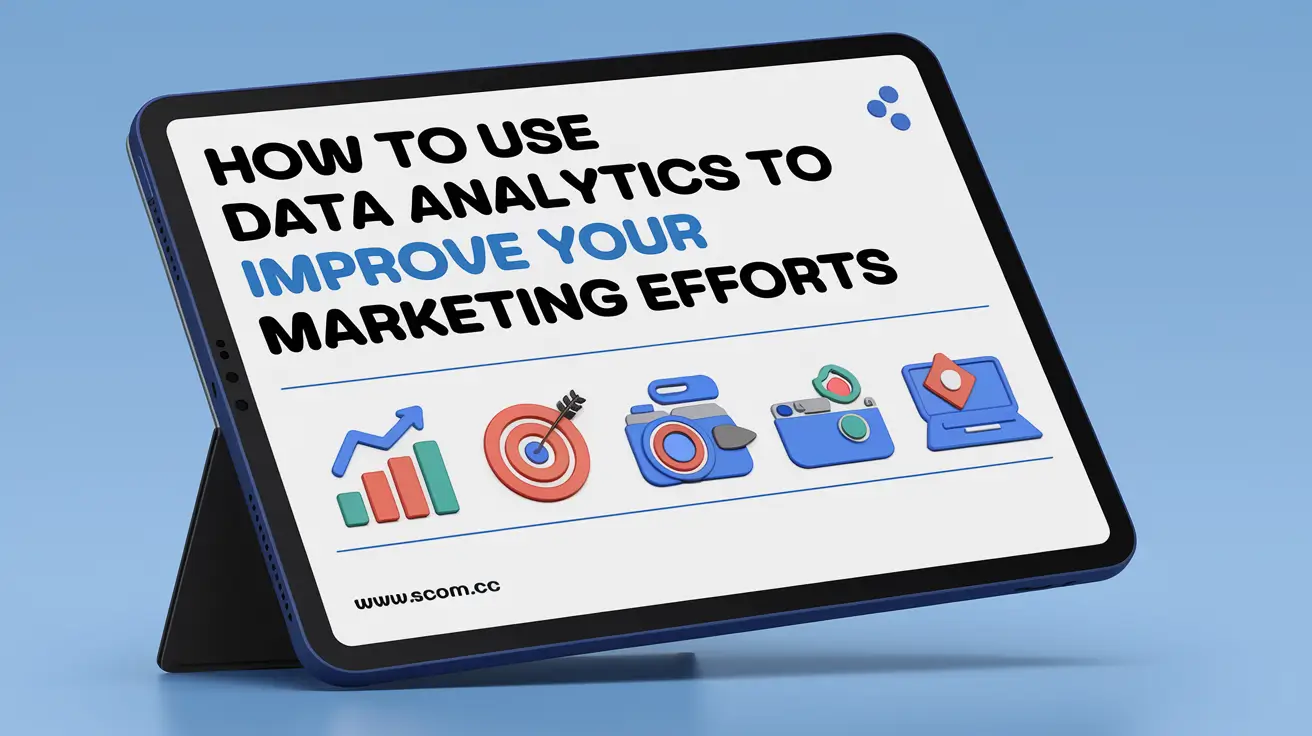
If you enjoyed this article and found it valuable, we encourage you to explore our news and valuable information section, where you’ll find more relevant and up-to-date content that may pique your interest. Additionally, if you are seeking advice or need guidance on a specific topic, we suggest visiting our services section. There, you will find a variety of options designed to assist and support you in addressing your needs. Feel free to check out both sections to get the information and assistance that best suits your requirements.